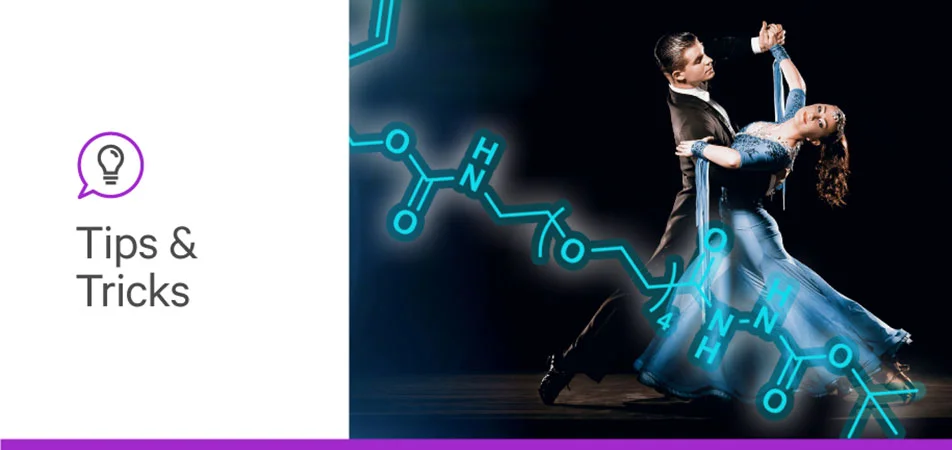
The idea of machines emulating human intelligence to perform tasks, make decisions, and improve their learning patterns was introduced to computer science in the 1950s [1]. Today, artificial intelligence (AI) is a highly-trending topic and a prominent part of our lives, from chatbots to digital phone assistants to smart homes. Its integration into our routine aside, AI plays a central role in life sciences, mainly biotechnology and bioinformatics, with the common goal of interpreting complex biological processes. AI algorithms are widely used to analyze big omics data to identify drug targets as well as to predict the activity of drug candidates on their targets.
Given that post-translational modifications, such as glycosylation, add a new layer of complexity to analyzing protein-protein and protein-drug interactions, the application of bioinformatics to glycobiology is necessary to understand and may predict the role of glycans in various forms of cellular behavior.
The implementation of AI for glycomics began in the 1990s with mass spectrometry pipelines, where machine learning algorithms were applied to predict glycopeptide fragment intensities [2]. With the increased emphasis on protein glycosylation patterns, researchers wanted to characterize glycosylation sites in more detail by studying the amino acid sequence of N-glycosylation and the lesser-studied O-glycosylation. Although it was known that glycan linkage occurred at the oxygen of a serine or a threonine, the role of the neighboring amino acids on O-glycosylation not been elucidated.
During the era of first-generation AI tools, datasets of glycosylation sites have been collected from proteins in tissue samples and biopsies, which were made available on databases such as UniPep [3] and N-GlycositeAtlas[4]. In addition, artificial neural network tools, such as NetNGlyc [5] and YinOYang [6] were developed to predict new N- and O- glycosylation sites using the known glycan data as training sets. Between 2005 and 2015, the predictive power of neural networks was improved through support vector machines and random forest algorithms. Based on these algorithms, software solutions like GlycoMine [7] used a multilayered prediction based on amino acid sequence, and structural and functional features of glycans to improve glycosylation site prediction.
Today, the influence of AI on glycobiology continues to expand with the combination of genomics, transcriptomics, and proteomics, as well as computational methods, which greatly enhance site prediction and glycan profiling. For example, Moon et al. developed a random forest algorithm that takes steric and electronic parameters of glycan stereoisomers to accurately predict the selective binding of a particular isomer [8]. Antonakoudis et al. used artificial neural networks in a systems-based approach, where a stoichiometric model was developed to predict glycosylation enzyme fluxes and the subsequent glycan abundances [9].
Meanwhile, other platforms, such as Glycowork, focused on processing broad glycan data to reveal organism-specific glycan profiles [10].
Besides site prediction and profiling, AI tools contributed to a better understanding of the complex relationship between glycans and cellular phenotypes. Qin et al. introduced an algorithm that uses single-cell SUGAR-seq data to predict the genes that led to N-glycan branching and the effect of different branches on T-cell subtypes in mouse models [12]. Interestingly, these genes were not uncovered in differential expression analysis between cell subtypes, which highlights the value of deep learning in phenotypic analysis.
Another exciting tool is GlyCompareCT, which – as its name suggests – compares the composition and abundance of glycan motifs in different datasets by decomposing them into glycan substructures [13]. This allows users to generate the complete set of motifs from the substructures. The Python-based nature of GlyCompareCT makes it a user-friendly tool that can be run via command-line.
While the multitude of glycoinformatics tools can contribute to our understanding of glycosylation, more work is needed to integrate next-generation machine learning into glycobiology. In particular, deep learning tools are instrumental when working with large and unstructured data sets. AlphaFold [14] is one of the pioneering projects that employs deep learning to predict protein structure, including its possible folded states. That said, the platform can only process protein sequences, thus lacking the foresight for glycosylation and other post-translational modifications.
More recently, deep learning methods began to be used for deducing glycosyltransferase structure and function from sequence data. Taujale et al. developed a workflow that used supervised deep learning to infer the folding state of glycosyltransferases from their protein sequences, which allowed them to predict their sugar donor specificities [15]. Subsequently, novel tools, such as GlyNet [16], SweetTalk [17], and glyBERT [18], began to emerge, with improved predictive value for the synthesis of branched and non-linear glycans. The same tools could also be applied to predict protein glycosylation sites [19].
One of the main challenges in glycobiology is the lack of broad glycomics data, which obscures the discovery of novel glycan structures. Next-generation AI models can overcome this issue by incorporating new features in addition to glycan structure. These features can be extracted from omics data that provide information about the upstream (e.g., precursor monosaccharides) and downstream processes (impact on signaling pathways). Since several glycans can share common synthetic steps or exhibit similar downstream effects, this knowledge can significantly enhance the scope of predicted glycans [20].
Finally, the consortium of machine learning tools can be leveraged to understand host-pathogen interactions. In particular, the ability to foresee cross-species transmission can help circumvent t he impact of future pandemics. Firstly, evaluating similar glycan structures across different species can reveal the host receptor-glycan interactions that allow viral entry to see which organisms are susceptible to viral invasion. It can also shed light on how pathogens use glycosylation to mimic host glycans to evade immune response. Furthermore, the combination of input, such as glycan similarity and phylogenetic distance – between humans and the animal studied – can inform us about the likelihood of pathogenic mutations that enable host switching towards humans. Preliminary models, such as SweetNet, leverage next-generation machine learning tools such as graph convolutional neural networks to identify glycan receptors on influenza and rotavirus while revealing binding specificities [21]. This approach can be extrapolated to several other viral proteins to explain how they are transmitted in humans.
Continuous development of AI models and integration of multi-omics could be invaluable for addressing various questions in glycobiology. These include but are not limited to glycosyltransferase structures, glycosylation sites on proteins, the impact of complex glycans on cellular function, pathogen-host interactions, and immuno-oncology (i.e., tumor microenvironment). The collection of novel insights gained from AI models will help researchers conduct more targeted studies to understand the role of glycosylation in health and disease.
There are currently many open-source software tools and databases on glycoinformatics. The Glycoinformatics Consortium (GLIC) webinar series is a great place to learn about some of these tools, particularly for storing and processing glycan array data. The most noteworthy microarray databases and processing tools include CarbArrayART [22], Glycan Array Dashboard (GLAD) [23], CarboGrove [24], and the Glycan Array Data Repository [25]. In addition, LectinOracle [26] and Glycowork [10] are promising deep learning-based tools to predict protein glycan interactions. A review article by Li et al. perfectly summarizes the collection of additional resources for the computational evaluation of glycosylation [27].
“To learn more about glycans and lectins and how they can be utilized in your workflow to push forward immunology research, check out our Exploring the World of Glycobiology ebook. For other resources and tips and tricks, stay tuned to the SpeakEasy Science blog. “
Stay in the Loop. Join Our Online Community
©Vector Laboratories, Inc. 2024 All Rights Reserved.